Putting together gendered datasets starts with leadership and a changed approach: looking at a specific problem that welfare wants to solve through a gendered lens. In its guidelines for integrating gender perspectives in national statistics, the UN defines gender statistics as “statistics that adequately reflect differences and inequalities in the situation of women and men in all areas of life.”
However, sex-disaggregated data are only the first component. The following criteria must be met before a dataset can be considered to contain gender statistics:
- Data are collected and presented by sex as a primary and overall classification;
- Data reflect gender issues;
- Data are based on concepts and definitions that reflect the diversity of women and men and capture all aspects of their lives;
- Data collection methods consider stereotypes and social and cultural factors that may induce gender bias in the data.
Adding a gender dimension to datasets is not simply a box to tick. Download the report to learn more.
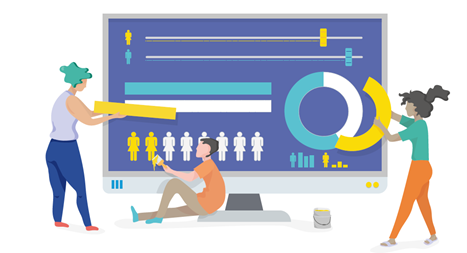
Gender mainstreaming has two components: gender analysis, which captures the status quo, and gender impact assessment, which evaluates the possible effects of a policy.
This gender analysis requires the creation of the gender-relevant datasets and statistics outlined above and an examination of the causes and effects of gender inequalities. For instance, a gender analysis of malaria not only captures quantified data about contagion, death, and recoveries, but also accounts for the risk incurred by pregnant women, the influence of gendered sleeping patterns and dress codes, or gender roles in polygamous families.
Gender impact assessment then considers qualitative criteria like the norms and values that influence gender roles, divisions of labour, and behaviours and inequalities in the value attached to gender characteristics. It also accounts for normative aspects, evaluating differences in the exercise of human rights and in the degree of accessto justice.
Starting gender mainstreaming from the policy planning phase is not only logical, but the timeliest and most cost-effective approach since it reduces the likelihood of problems in the implementation phase. Download the report to learn more about the problematic implementation of discriminatory ADMS in three Western countries.
Since ADMS follow human psychologies and prejudices, many pitfalls can be avoided by co-designing the rules they should apply and the interfaces that these systems use to interact with claimants.
Furthermore, human oversight is fundamental in avoiding the automation of errors and inequalities. Precisely because of their binary reasoning for which they are perceived as neutral, ADMS cannot capture or react to the countless nuances of every welfare claimant’s case.
The experience of caseworkers could also be instructive, as they deal with claimants face-to-face, and are used to bending the rules when necessary. Digital welfare should build entry points for caseworkers in ADMS and improve their interactions with the systems instead of replacing them altogether.
Of course, with welfare systems there are real life consequences to errors and misjudgements made by ADMS. In rejection cases, citizens may only find out when it is too late and therefore need agency over their own rights and should be able to exercise those rights in the digital world. Interactions with system should be possible at any step of the process, and these should not be left to chatbots or other forms of AI. Even the opaque way in which the interaction is designed can expose claimants to undue stress, particularly when digital divides are taken into account.
“Explainability is useless without the concept of understanding: the process needs to be understandable. Moreover, there needs to be visibility of how and where the system is being used, people are not aware.”
– Dr. Rumman Chowdhury, Accenture
The previous principles facilitate the automation of a certain degree of equality. The system must consider data that are representative of the situation in order to support decisions based on gender-responsive policies, as well as interface with frontline workers and citizens in an accessible and understandable way. The “fairness” of an ADMS would not be intentional, but rather the result of better processes behind its creation.
“There is a great disconnect between historical reality and desired reality. If you are just going to pull in more historical data about any disadvantaged group, you run the risk of more strongly embedding their historical disadvantage. You need to push the system towards a desired scenario.”
– Dr. Angsar Koene, University of Nottingham
These principles can from the basis of design exercises that can reverse-engineer situations of desire, in effect automating the overturning of historical discrimination and systemwide biases. Encapsulated in the notion of welfare is the mission of improving the lives of vulnerable individuals, families and communities. Women have been historically treated as passive agents, often relegated to the domestic private space. Embedding a profound understanding of women’s actual conditions into the design processes behind ADMS offers revolutionary potential to transform welfare systems.